Incrementality Testing Series Episode #2: Conversion Lift Study
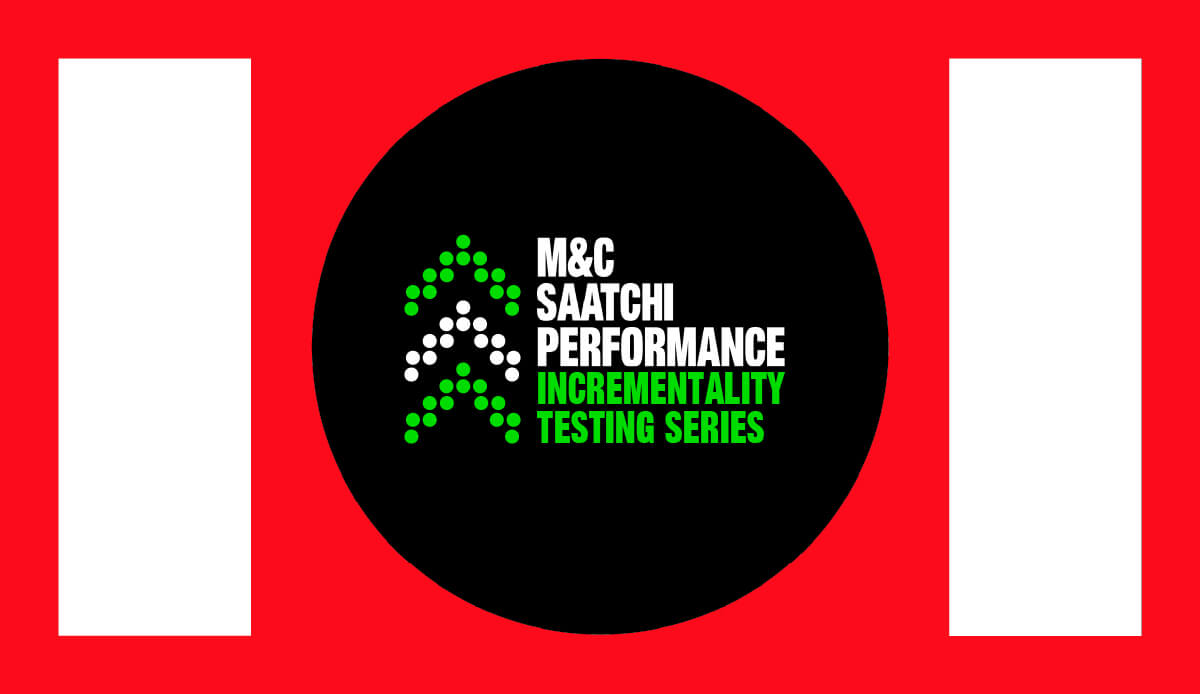
Welcome to part two of our three-part series on incrementality testing. In this piece, we take a closer look at conversion lift testing and its growing importance within the media industry.
As we explored in part one, we are stepping into a privacy-centric, cookieless world – a reality already materialized on certain platforms. This change sounds the death knell for deterministic attribution, a methodology traditionally reliant on an abundance of user-level data. However, one thing that is not changing is the way we consume media – in fact, if anything, our consumption will become more digitally skewed in the coming years.
We are therefore faced with an interesting problem: digital channels becoming more important for brands, alongside a measurement ecosystem that has exploded in complexity, with traditional approaches no longer being fit for purpose. It’s crucial to remember, however, that while measurement will become more difficult, and will present a new and unique set of obstacles, it is far from impossible. The key lies in a subtle shift in how we think about performance and the tools we use to measure its impact.
This is where incrementality comes into play.
The goal of an incrementality test, as its name suggests is to calculate the incremental value (or lift) driven by an ad/ campaign. It measures the true impact of your campaign on business outcomes (installs, trials, revenue etc). By testing different channels and campaigns separately – e.g., Facebook, TikTok, Twitter – we can then compare incremental impact/ lift, like-for-like, across each channel and optimize our budgets accordingly.
“Incrementality tests depend on careful pre-test planning to yield meaningful results. In particular, it is crucial to set realistic expectations about the potential uplift and, consequently, the budget required to achieve statistically significant and trustworthy outcomes.”
BEN REID
ANALYST, DATA ANALYTICS & TECH,
M&C SAATCHI PERFORMANCE
Conversion Lift Study – Test Design
A conversion lift study (or lift test) follows a nearly identical methodology to brand lift studies, something I’m sure we’re all familiar with.
It is a Randomised Controlled Trial (RCT)1 that divides a channel’s audience into two groups: the test group that is exposed to the marketing campaign/ad, and the control group (aka holdout) that does not see the ad. Outcomes/ conversions are then compared between the two groups – if the test group drives more outcomes/ conversions than the control group, the difference between the two groups is called your lift, and is the incremental impact attributed to the ad.
RCT (Randomised Controlled Trial): a test in which each subject taking part in the experiment is randomly assigned to either the test group (exposed to the ad) or the control group (not exposed to the ad). The two groups are tracked and their conversion are recorded. We then analyze the results using statistical methods to understand if the ad exposure drove more conversion.
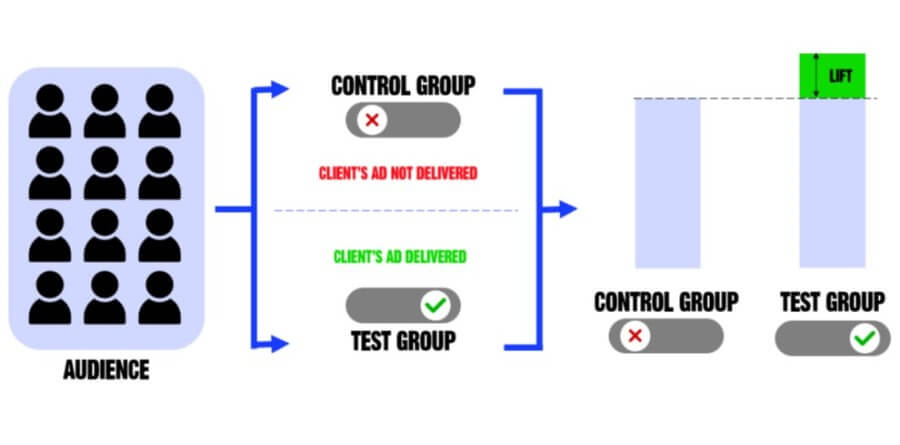
Conversion Lift Study – Example
Let’s look at an example: Brand A rolled out a new campaign on Facebook and decided to evaluate its impact through a conversion lift test. Their audience was split into two groups:
- Control Group: Comprised of 7,000 users who did not see the ad, resulting in 700 conversions.
- Test Group: Another 7,000 users who saw the ad, with 1,000 conversions recorded.
After collating the results, the difference between the control and test groups’ performance is examined for statistical significance. This can typically be delivered as follows:
- Not Statistically Significant: This might occur due to a variety of factors, with the implication being that the results may not be entirely reliable. In conversion lift testing, a common issue is an insufficient budget. This requires a rerun of the test.
- 80% to 90% Confidence Interval: Results falling within this range can be used for directional planning. However, conducting another test to confirm these results may be beneficial.
- Above 95% Confidence Interval: These results are deemed statistically robust. This means that if we repeated this process, we would expect the true impact of our media to fall within our estimated range about 95 times from 100. Results at this confidence level are reliable enough to be used for planning and optimization.
For the sake of our example, we’ll assume that Brand A’s test results were significant, with a confidence interval of 95%. Lift is usually reported in two ways:
- Relative Lift: This refers to the percentage increase in conversions for the test group compared to the control group. It is calculated by dividing the difference in conversion rates between the test and control groups by the conversion rate of the control group.
- Absolute Lift: This term refers to the raw difference in conversion rates between the test and control groups.
In Brand A’s case, the control group’s conversion rate was 10% (700 conversions / 7,000 users), while the test group’s conversion rate stood at 14.29% (1,000 conversions / 7,000 users).
- Relative lift was calculated as ((14.29% – 10%) / 10%) * 100%, resulting in 42.9%.
- Absolute lift was a simple subtraction: 14.29% – 10%, yielding 4.29%.
This data tells us that Brand A’s campaign resulted in a relative lift of 42.9% and an absolute lift of 4.29%. Given the 95% confidence interval, these robust results are invaluable for refining campaign planning and optimization
Conversion Lift Study – Current Challenges
While the concept of conversion lift study is simple and straightforward, it isn’t without its challenges, particularly in a world where third-party tracking is depreciating. There are two key challenges here: having enough conversion volume to generate statistically robust results, and accurately measuring the conversion volume from the control group – the group that did not interact with the ad. For instance, at the moment, TikTok only provides conversion lift studies for Android users.
Like with any test, we want to ensure the observed lift is predominantly due to the marketing campaign being tested. Therefore, minimizing the media spend outside the test during the experiment is crucial. This helps to reduce bias and confirms that only the users who see the ad are in the test group.
Despite these challenges, implementing conversion lift tests is still possible, although they are increasingly becoming more complex.
So, What’s Next ?
To overcome this challenge, another solution has been developed that could assist marketers and media agencies: geo/matched market tests. These tests are immune to any changes in tracking/ data privacy and are considered the gold standard of incrementality testing in a privacy-centric world. Part Three of this series will be a deep dive into geo/ matched market tests, what’s needed and what they look like in practice.
Key Takeaways:
- Measurement is evolving. The disappearance of user-level tracking adds complexity, but it doesn’t make measurement impossible. It does, however, necessitate a shift in our approach to performance assessment.
- In a privacy-centric world, incrementality testing is a crucial tool for marketers. It allows for the measurement of the true impact of media campaigns on business outcomes, enabling continued media optimization.
- Conversion lift tests are considered the gold standard for media testing. They require the ability to split audiences at an individual level and therefore can only be conducted within a platform.
- Lift is typically reported in two ways: relative and absolute. Relative lift represents the percentage increase in conversions for the test group versus the control group, while absolute lift indicates the raw difference in conversion rates.
- Pre-test setup is vital when planning and setting up your test. It’s highly recommended to collaborate with your analytics team to ensure an optimal setup, thereby maximizing the chances of obtaining actionable results.